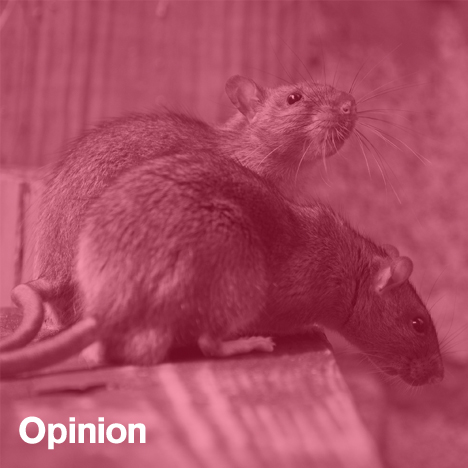
"A city is more than just the sum of its previous behaviours"
Opinion: from Chicago's rat problem to transport startups like Bridj and Uber, data is being used to predict behaviour and manage our cities. But the idea of the "Predictive City" should be treated with a healthy dose of scepticism, argues Dan Hill.
John Lanchester tells a story in How to Speak Money – a book I recommend to any designer or architect wishing to understand the context their work is produced in — about the way Ancient Egypt worked. In short, everything hinged on the annual inundation of the Nile flood plain: the society itself, arguably the most stable the world has ever seen, its cultural artefacts, such as their calendar, their understanding of seasons, their taxation system, and of course their agricultural cycles — all were directly linked to the Nile's flood. The priesthood of Egyptian society, drawing from rich mythologies, performed complex rituals to divine the nature of the flood, and thus the harvest, each year.
But Lanchester reveals how the priests actually did it: they were cheating. Unbeknownst to the population, they had a Nilometer, a device to predict the level of flood water. Based on measuring stations secreted in temples, the Nilometer captured the flow of the river, plotted it against various markers and – combined with flood records dating back centuries – enabled relatively accurate predictions of that year's harvest. Lanchester suggests "the Nilometer was an essential tool for control of Egypt. It had to be kept secret by the ruling class and institutions, because it was a central component of their authority."
Not that Herodotus would've put it like this, but this is perhaps the first example of predictive analytics in urban governance.
Predictive analytics is the ability to deliver services for future events, before the need has manifested itself, based on the accretion of "big data" about past events. Although urban planning and policy has always been a form of prediction — sometimes combined with agency to make it true — this is an order of magnitude shift in data gathering and number crunching; and so, in turn, in the purported accuracy of what can be predicted.
The same principles are in play across an increasingly wide range of services. Based on the data they have, Amazon can apparently guess what you're about to buy before you know you want to buy it, and will move it nearby accordingly (they filed a patent for "anticipatory shipping" earlier this year).
Yet when people talk about predictive analytics and cities, they often turn to Chicago's rats. Combining many previously disparate data sets, such as calls to the city's 311 services related to garbage or broken water mains, over geospatial databases — AKA maps — has enabled the city to predict where infestations are likely to occur before they do. Just as Mr. Rat has paid the deposit on a new place and picked up his keys, he turns the corner to find a Chicago Department of Streets and Sanitation officer leaning against the door, whistling.
This idea, and its impacts, could fundamentally change the way we think about running cities generally. Using similar techniques, and digging ever deeper into urban data, Chicago could soon predict which buildings are most likely to catch fire next, where vacant properties will occur, or where planning violations might be likely. More controversially, Chicago's datasets include a "heat list" of those 400 or so individuals in the city that are most likely to be involved in a violent crime — it enables police to pre-emptively, well, "address" those on the list, which some see as an exaggerated extension of Stop-and-Frisk.
The "heat list" has come under much scrutiny in Chicago (and LA and New York, where similar software has been deployed) but few realise that these systems are increasingly in use everywhere, from Seattle to Kent. Here the difference between pest control and policing should be clear, but you can imagine the attraction to a certain kind of politician — "Sir, you can eradicate crime, nipping it in the bud before it happens" — irrespective of the enormous ethical, and indeed constitutional, issues. Once the list exists, it's difficult to put that particular genie back in the bottle. What if you don't act upon the list and someone dies? Equally, what if you do act upon the list? And how exactly do you do that?
Perhaps less immediately contentious is the use of predictive algorithms in public transport. If transport agencies can scrape data from the surfaces of their city's interactions, they can build models of behaviour that enable them to predict where the demand for transport is needed, before anyone asks for it. In other words, instead of walking to the bus stop, the bus stop comes to you.
These dynamics underpin Bridj, a transport startup in Boston. Bridj uses patterns of transport use, combined with social media analytics, in order to send its fleet of buses to where there is demand for a fleet of buses — on the fly. It is largely post-timetable, post-route. It could sit neatly between mass transit and private car ownership, working as a form of "relief valve" for the Boston MBTA.
Where have we seen on-demand transit before, outside the limited horizons of US tech culture? Interestingly enough, many of the more useful reference points for these developments occur in more informal urban environments.
Designer Keiichi Matsuda tells me about the gloriously-festooned buses that careen around Medellin, essentially occupying a legal grey area as well as often unpredictable street routes, and sometimes, the pavement. In Nairobi, the equally gaudy matutu buses are fighting off Google NFC-enabled smart cards, preferring to transact in cash — whilst matutu may observe a form of bus stop, they move through traffic at full pelt as if autonomous vehicles (though with a rather different safety record). Even highly-regulated cities like New York have what The Verge called "a shadow transportation network" of "dollar vans" and buses, serving areas like Chinatown, or particular communities. Often unlicensed, sometimes the police apparently turn a blind eye, and even welcome their presence; other times, not so much.
These services fill in the cracks and gaps of the formal transit networks in a broadly similar way to Bridj, yet based on driver knowledge, instinct and small data, if we can call it that, about local culture. The fact they don't scale doesn't really matter, and in some senses they are more legible, local and, well, likeable than an Uber, say.
Yet both the matutu and the metro could be derailed by startups like Bridj, Lyft, Uber, Relayrides et al. None of these startups have a primary aim of putting public transit agencies out of business, yet the adjacent space they play in could be close enough to destabilise those incumbent agencies — just as Airbnb is not officially in the hotel business, yet clearly impacts upon it. Startup culture plays by its own rules, and is a little careless and untidy about how it does so.
More broadly, predictive analytics applied to crime could cause the rate of violence in a city to plummet — yet might the way it does this also shred social fabric? These implications are adjacent, slippery, opaque, and laden with assumptions about the positive effects of data-driven services on the city.
Uber says that regulations, generally, are outdated, as they were written before we carried smartphones around. This is true. The question is what one does about it, and further, what ideologies underpin such decision-making.
An excited Harvard Business Review notes that "It's still pretty amazing that we can use analytics to predict the future. All we have to do is gather the right data, do the right type of statistical model, and be careful of our assumptions."
Be careful of our assumptions, indeed. Whilst the notion of "path dependency" obsesses urbanists, a city is more than just the sum of its previous behaviours; just as a former violent criminal might not ever be violent again.
Fundamentally, with this Predictive City in mind, the sheer unpredictability of cities is not only part of their charm, but a vital lesson about the possibility of change. As Oscar Wilde said, "every saint has a past, and every sinner has a future." Predictive analytics, if applied with a carelessness Lady Bracknell would recognise, has no time for such subtleties.
Such approaches needn't necessarily be detrimental — they could be highly informative, in the context of more holistic, collaborative, imaginative approaches to designing and running cities. But only if, unlike the priests of Ancient Egypt and their secret Nilometers, we are openly discussing the potential pitfalls — the possibility that ironing out unpredictability also irons out difference, and the possibility of change itself — and sketching out richer versions of our urban future.
Dan Hill is executive director of futures at Future Cities Catapult. He is an adjunct professor in the Design, Architecture and Building faculty at University of Technology, Sydney, and his blog City of Sound covers the intersection between cities, design, culture and technology.